Datasets of relevance¶
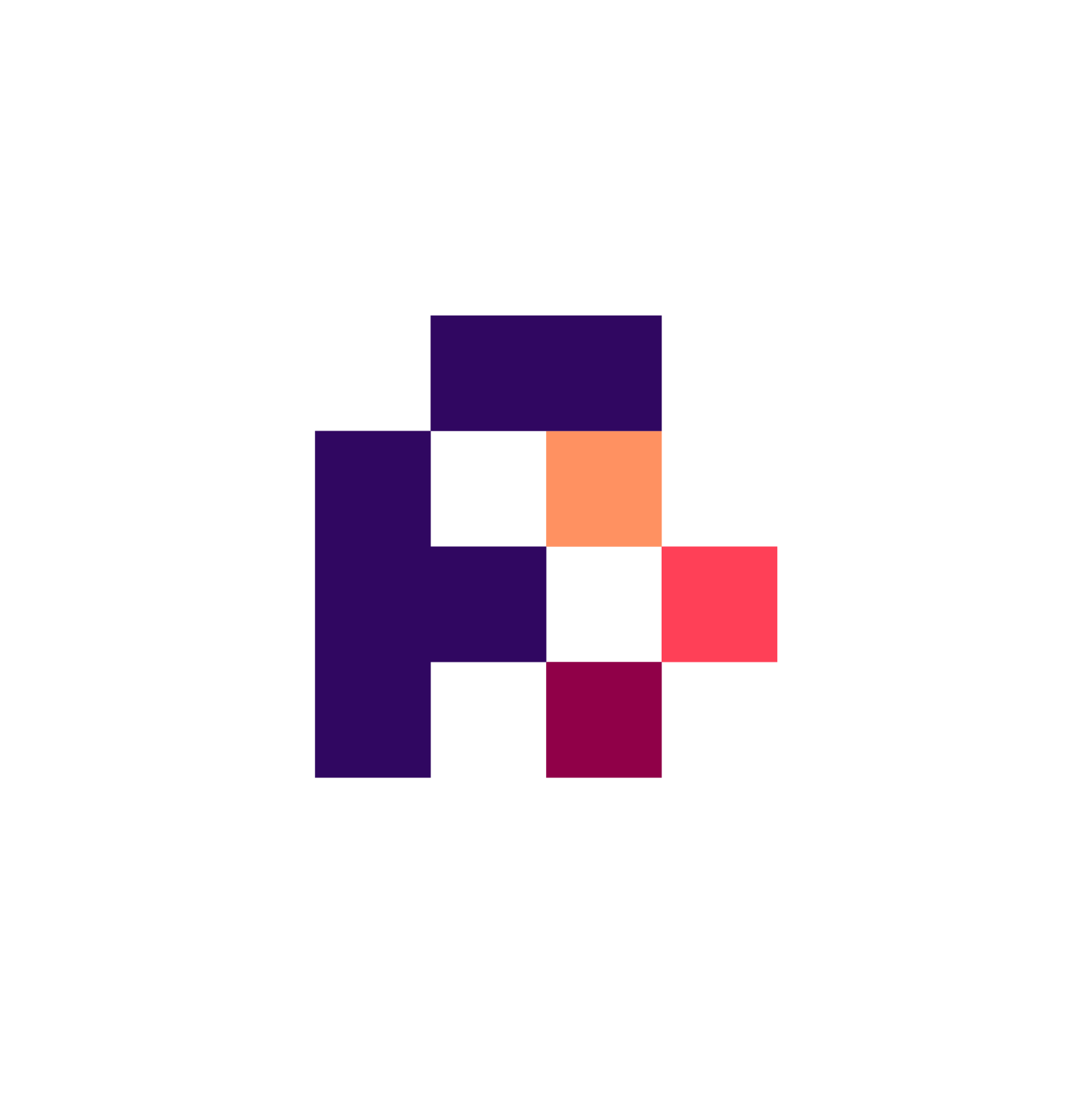
Main Objectives¶
The FAIR cookbook aims to provide hands-on, practical advice on how to deliver FAIR data through interactions with Innovative Medicine Initiative projects. These research projects, by nature often involve patient-centric information but dealing with real-world data and human-centric information, clinical data, in particular, is challenging. It most often mandates interacting with DACs, i.e. Data Access Committees, and undergoing a vetting process, which can be lengthy and convoluted. This can become a hindrance if the focus of the work is to deliver training on the computational methods available to deal with such data rather than data custody-related tasks, however important these are.
This FAIR cookbook recipe aims to provide a list of relevant resources belonging to the realm of clinical data so readers can, with the minimal hassle :
familiarize with the data types (for instance, how do Electronic Health records look like).
familiarize with the procedures to gain access to sensitive data.
obtain datasets with which to work and hone computational skills.
The recipe will cover two types of datasets:
real datasets
such as the MIMIC-III dataset 2, which corresponds to actual medical notes data for which data access requests must be made but which are made available to computational scientists for research purposes.synthetic datasets
, which are available without restrictions since produced by computational methods and independent of any real patient. While handy, this type of data may come with a number of limitations prospective users need to be aware of.
Electronic Health Records: The MIMIC-III Critical Care Database¶
Data Type: Electronic Medical Notes
Nature of the data: Real Patient Data
Availability: Requesting Access:
https://mimic.mit.edu/docs/gettingstarted/ https://mimic.physionet.org/gettingstarted/access/From Amazon Web Service Public Data: https://registry.opendata.aws/mimiciii/
Description: MIMIC-III (Medical Information Mart for Intensive Care III) is a large, freely-available database comprising de-identified health-related data associated with over forty thousand patients who stayed in critical care units of the Beth Israel Deaconess Medical Center between 2001 and 2012. 2. The database includes information such as demographics, vital sign measurements made at the bedside (circa 1 data point per hour), laboratory test results, procedures, medications, caregiver notes, imaging reports, and mortality (both in and out of hospital).
Purpose: MIMIC supports a diverse range of analytic studies spanning epidemiology, clinical decision-rule improvement, and electronic tool development. It is notable for three factors: it is freely available to researchers worldwide. It encompasses a diverse and very large population of ICU patients. it contains high temporal resolution data including lab results, electronic documentation, and bedside monitor trends and waveforms.
Format: SQL database dump
License: https://physionet.org/content/mimiciii/view-license/1.4/
Examples of use:
Electronics Medical Notes: The EBM NLP¶
Data Type: Electronic Medical Notes
Nature of the data: Synthetic Data
Description: A corpus containing 4,993 abstracts annotated with
(P)articipants
,(I)nterventions
, and(O)utcomes
.
Training labels are sourced from AMT workers and aggregated to reduce noise. Test labels are collected from medical professionals. 1
Format: ad-hoc, UTF-8 text file with tab delimited values
Availability: https://github.com/bepnye/EBM-NLP
Purpose: Corpus for training and testing Natural Language Processing algorithms
Data Type: Electronic Medical Notes
Nature of the data: Synthetic Data
Description: This test data set, generated by Lee Evans while working at LTS Computing LLC is an OMOP-CDM version 5 formatted collection of 1000 patient sample of CMS 2008-2010 Data Entrepreneurs’ Synthetic Public Use File (DE-SynPUF). The information held in the dataset corresponds to Synthetic patients & medicare claims/prescription data. he dataset can be downloaded in a compressed (zipped) format from LTS Computing LLC website: http://www.ltscomputingllc
Format: OMOP CDM v5.2.2
Availability: http://www.ltscomputingllc.com/downloads/
Purpose:
Public data to demo the OHDSI Ontologies
Benchmark performance
Developing & testing OHDSI tools
OHDSI tools training
License: LTS Computing LLC license
Synthean Electronic Health Records¶
One of the main bottlenecks for data miners is the lack of dataset availability of electronic health records, due to, as we saw it to HIPAA concerns. To bypass these roadblocks, several tools have been developed to generate synthetic datasets, free of any restrictions. Below, we provide information about one such tool.
https://github.com/synthetichealth/synthea/wiki
Data Type: Electronic Health Records
Nature of the data: Synthetic EHR
Description: Synthean, Synthetic Electronic Health Records generating software 3
Format: HL7 FHIR
Availability: https://synthetichealth.github.io/synthea/ https://synthea.mitre.org/downloads
Purpose:
Generation of synthetic training dataset for data mining and algorithm development
License: CC0 - “Public Domain Dedication”
Exemplar datasets available for download
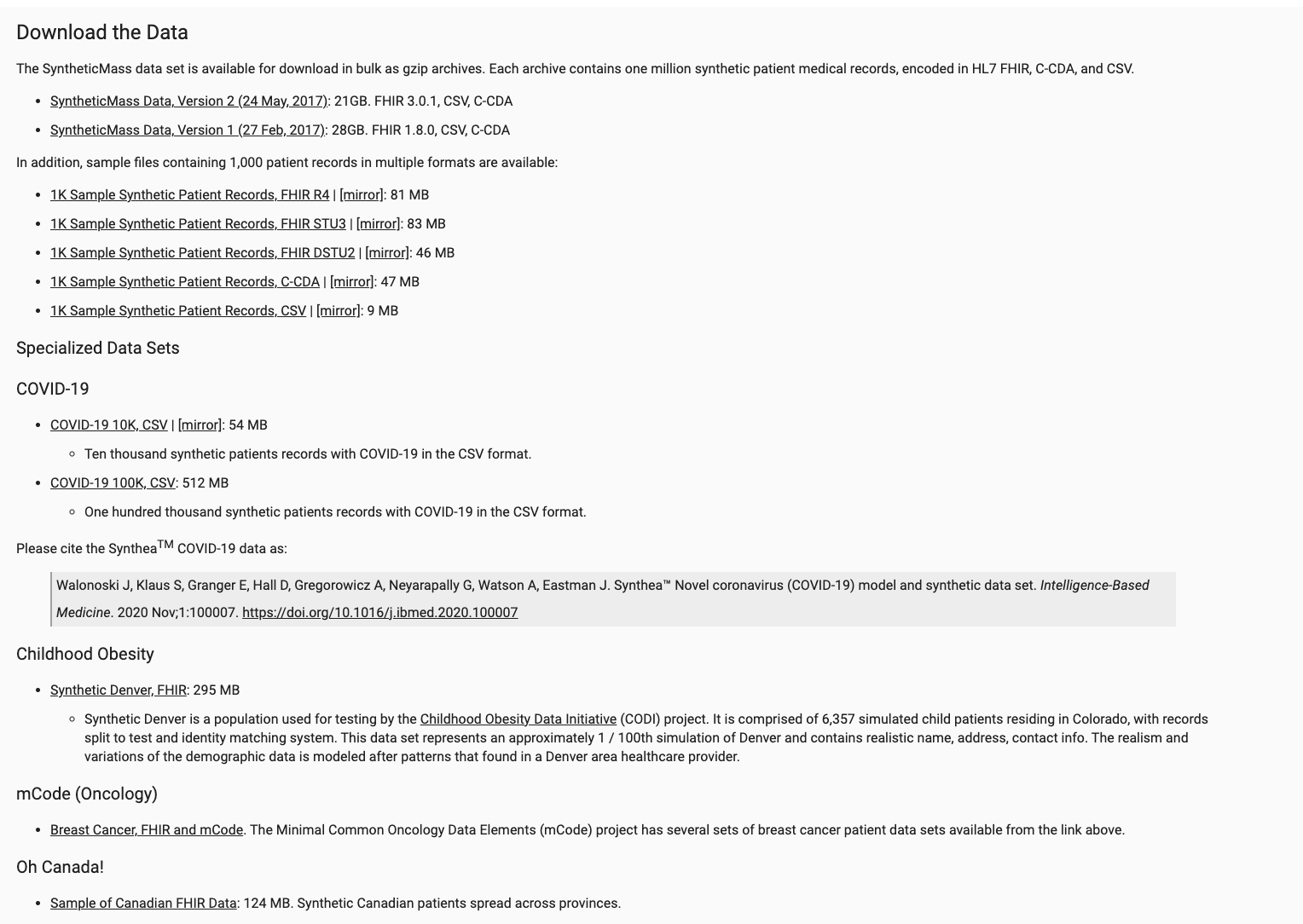
Fig. 11 Exemplar Synthetic EHR datasets available for download in HL7 FHIR¶
Synthetic Electronic Medical Notes: the OMOP CDMv5 Test Data¶
Data Type: Electronic Medical Notes
Nature of the data: Synthetic Data
Description: This test data set, generated by Lee Evans while working at LTS Computing LLC is an OMOP-CDM version 5 formatted collection of 1000 patient sample of CMS 2008-2010 Data Entrepreneurs’ Synthetic Public Use File (DE-SynPUF). The information held in the dataset corresponds to Synthetic patients & medicare claims/prescription data. he dataset can be downloaded in a compressed (zipped) format from LTS Computing LLC website: http://www.ltscomputingllc
Format: OMOP CDM v5.2.2
Availability: http://www.ltscomputingllc.com/downloads/
Purpose:
Public data to demo the OHDSI Ontologies
Benchmark performance
Developing & testing OHDSI tools
OHDSI tools training
License: LTS Computing LLC license
SynPUF 1000 person dataset in OMOP CDM v5.2.2 format:
This synthetic dataset corresponds to 1000 person composite dataset:
http://www.ltscomputingllc.com/wp-content/uploads/2018/08/synpuf1k_omop_cdm_5.2.2.zip
For more information about the OMOP Common Data Model, refer to the following:
CDM 5.2.2 DDL for the OHDSI supported DBMSs is available health-related
Clinical Trial Data in CDISC SDTM format:¶
Data Type: Clinical Trial Data
Nature of the data: Dummy Trial Data
Description: This is a sample study dataset containing CDISC SDTM formatted data files created originally by CDISC for demo purposes. This dataset can be used by anyone who is interested in CDISC SDTM formatted dataset. This version is used to demonstrate loading standard clinical datasets into PlatformTM: a data custodianship platform for translational research data. Live demo here: https://platformtm.cloud/app/#/projects/15/main
Format: CDISC SDTM
Availability: https://dataverse.harvard.edu/dataset.xhtml?persistentId=doi:10.7910/DVN/51B6NK
Purpose:
Benchmark performance
Developing & testing CDISC tools
CDISC SDTM tools training
License: CC0 - “Public Domain Dedication”
Observational Data in OMOP CDM format:¶
Data Type: Observational Data
Nature of the data: Dummy Trial Data
Description:
Format: OMOP CDM v5
Availability:
Purpose: *
License: CC0 - “Public Domain Dedication”
Conclusions¶
This content provides you with a set of resources to kick start your exploration of unstructured text in clinical context. These are useful resources for gaining familiarity with these data types. Remember to understand the data stewardship requirements that go along with handling real clinical data but also the limitations associated with some synthetic datasets.
What should I read next?¶
How to request data access and deal with data access committees?
How to do NER on EHR with NLP?
How to deal with unstructured text?
References¶
Reference
- 1
Cdmv5 test data. URL: https://www.ohdsi.org/wp-content/uploads/2015/04/Lee_Evans_CDMV5_Test_Data_Presentation.pdf.
- 2(1,2)
A. E. Johnson, T. J. Pollard, L. Shen, L. W. Lehman, M. Feng, M. Ghassemi, B. Moody, P. Szolovits, L. A. Celi, and R. G. Mark. MIMIC-III, a freely accessible critical care database. Sci Data, 3:160035, May 2016.
- 3
J. Walonoski, M. Kramer, J. Nichols, A. Quina, C. Moesel, D. Hall, C. Duffett, K. Dube, T. Gallagher, and S. McLachlan. Synthea: An approach, method, and software mechanism for generating synthetic patients and the synthetic electronic health care record. J Am Med Inform Assoc, 25(3):230–238, Mar 2018.
Authors¶
Authors
Name |
ORCID |
Affiliation |
Type |
ELIXIR Node |
Contribution |
---|---|---|---|---|---|
University of Oxford |
Writing - Original Draft |
||||
University of Oxford |
Writing - Review & Editing |
||||
Fraunhofer Institute |
Writing - Review & Editing |